The House of Data Integrity Compliance
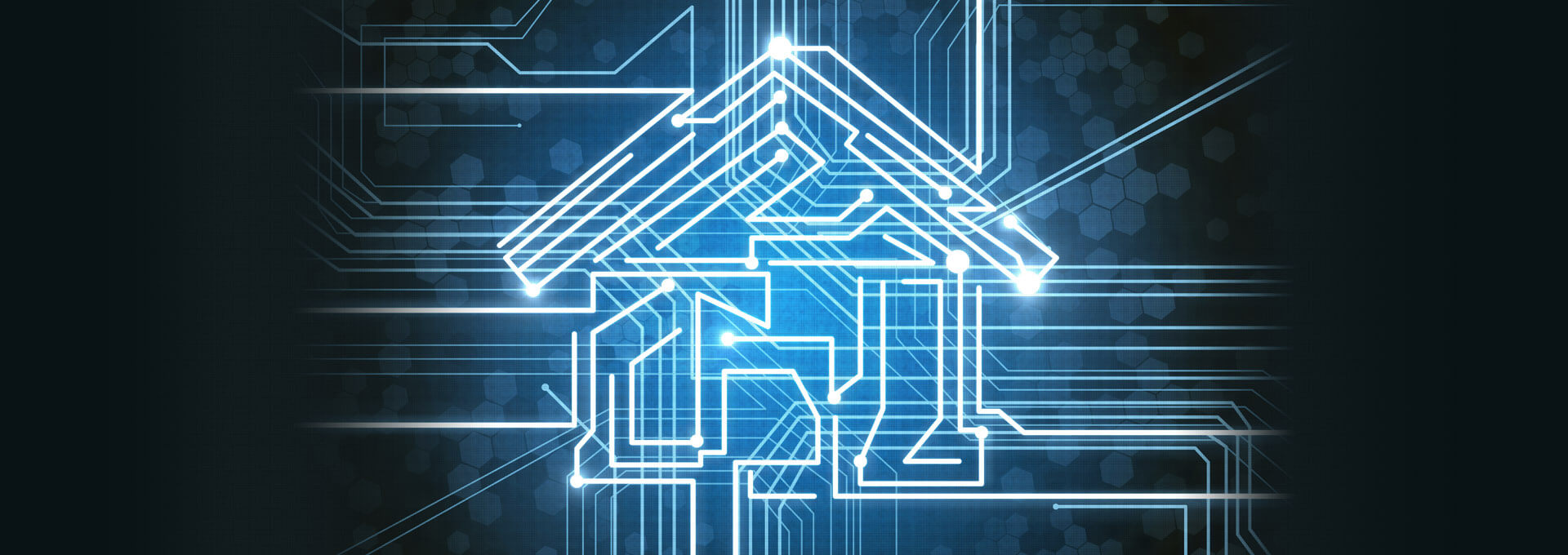
Various industry influences have challenged how we—as scientists, manufacturing technicians or quality control professionals—approach the processes that protect the integrity of the data we collect during the manufacture and release of products that impact human and animal health globally.
Factors like the shift to more computer-interfaced processes in the laboratory and the manufacturing suite necessitated the shift from paper-based data collection systems to any combination of paper/electronic data collection systems. This shift affects all areas of the product lifecycle, from raw material to commercial product release and maintenance.
Visualization is often used to aid teams in creating a structured methodology for problem-solving or illustrating key targets for program success. With data integrity, the intent is to enable conditions that drive systematic control of the creation, storage conditions and access to records and data that lead to important decisions about product quality and patient safety. These key points can be organized into what is called the “house of data integrity compliance.”
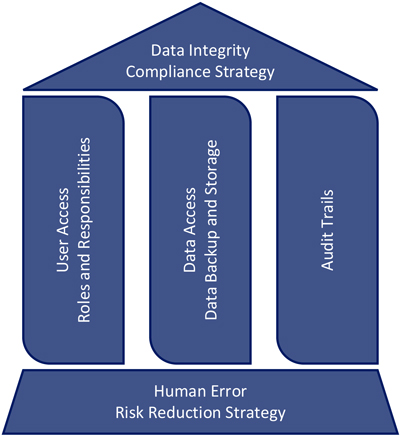
The foundation of the house is constructed of the strategies that enable an organization to reduce or completely mitigate the risk for human error that occurs in their processes. Each beam of the house represents the specific software and electronic strategy for compliance with data integrity regulations and guidances.
These collective factors provide the support structure for compliance—the roof of the house—and complete the house of data integrity compliance.
House Foundation: Human Error Risk Reduction
Human error risk reduction strategies provide an important tool for organizations to evaluate their data integrity compliance stance and provide avenues to improve product quality. Research from many industries shows that mitigating the risk for human error is not related to analysts lacking the knowledge, skill or ability to perform a task, but rather correcting the processes that allow for errors to occur in the first place (1). As an example, let us look at the assays and processes carried out in the typical microbiology laboratory. These processes tend to be based on methods that are antiquated in the face of current GMPs and involve a layer of subjectivity that forces the manufacturing or laboratory analyst to make a judgment call in order to generate data. Good examples of this are the gel-clot assay for endotoxin detection, gram-staining for microbial identification and counting colonies on a plate for the detection of bioburden in the manufacturing process.
Recent research in the airline manufacturing industry has gone a long way to categorize the different types of human error and common mitigation strategies; these same strategies can be used in the pharmaceutical industry. Processes that rely on subjective judgment calls to generate data tend to necessitate strategies to mitigate the risk for human error that fall into a category called “duplication.” This strategy involves using a second analyst to make the same read for every sample after the first analyst; both reads are then checked for agreement with each other. Employing this strategy to ensure performance of a subjective process is the least-effective method in reducing human error-related failure modes.
On the other end of the spectrum is updating or changing the process in some way as to remove the subjectivity that allows for human error to occur at all. This strategy falls into a category called “error proofing,” which typically involves automating part or all of the process and is the most effective strategy for eliminating the risk for human error in any process. A reduction in human error has the added benefit of making processes more efficient and more effective, leading to more impactful data generation. Representative data and increased process knowledge allow organizations to transform into more effective decision-makers in terms of product quality and data integrity compliance.
House Beams: Software and Electronic Data
The house beams constitute a strategy for continual improvement and validation of three key components of software and electronic data integrity compliance:
- User access and roles and responsibilities of specific users
- Data access and data storage and backup
- Compliant, consistent and complete audit trails
Control of these processes is a key indicator of the risk to noncompliance with regulations that provides a health check of what methods and strategies an organization has in place to mitigate or eliminate that risk. Restricted user access protects against unauthorized access to the critical data that determines product quality. Defining the roles and responsibilities of each user ensures that a system of checks and balances is in place to further limit access to data based on pre-defined internal knowledge levels. Data backup provides organizations with a route of recovery in the case of an emergency or a disaster. These strategies also become critical during justification of decisions to all key stakeholders, including in audit situations. Last, but not least, accurate and complete audit trails allow an organization to catch anomalies in data generation and backup. An audit trail provides a roadmap of events that enable organizations to investigate root causes, recover, and close gaps that present a risk to the data they depend on to make manufacturing and quality decisions. Although not a complete overview of data integrity risk, these three components provide a structure for organizations to standardize their electronic data integrity compliance strategy.
Taken together, the key components that make up the house of data integrity compliance provide the building blocks of a successful compliance strategy. Reducing the risk for human error translates into the production of higher quality data in both manufacturing spaces and the laboratory, while also ensuring efficient and effective electronic and automated processes. A well-defined electronic data integrity strategy ensures that access to critical data is controlled, that data is protected, and that the organization is able to recover from an emergency or failure mode. A strong data integrity compliance strategy yields the highest product quality and ensures safe products and healthy patients.
The availability and cost-effectiveness of automated systems coupled with electronic data collection platforms have made the paper-to-electronic-data capture shift even more attractive for organizations looking for the right balance of efficiency and cost, while increasing product quality and confirming patient safety. The wide availability of data has also spurred initiatives like the adoption of LEAN and Six Sigma principals that focus on making data-driven and scientifically sound decisions about the processes that take place in the manufacturing space and laboratory. Collectively, these factors have impacted our interpretation of data integrity expectations and regulations so that a focus on patient safety is at the forefront of every debate, conversation or regulatory citation.
In December 2018, the U. S. FDA published an updated, comprehensive guidance for industry, in the form of a question and answer document, on current GMP and compliance with data integrity regulations in the laboratory and beyond (2). Regulatory authorities require the data we collect during the manufacture of drugs and medical devices to be reliable, relatable, representative and accurate. The challenge given to us as an industry is to define sound strategies that rely on representative data and to drive a quality culture that can recognize and mitigate the risk for data integrity lapses. The data we collect in the manufacturing and laboratory spaces is critical to confirming process control, product quality and, most importantly, patient safety. Therefore, it is a critical part of our jobs to ensure that this data is attributable, legible, contemporaneous, original, and accurate (ALCOA).
References
- Reason, James. 2000. “Human error: models and management.” BMJ 320 (7237): 768-770. https://doi.org/10.1136/bmj.320.7237.768 (Accessed Aug 6 2020)
- U.S. Food and Drug Administration. 2018. “Data Integrity and Compliance with Drug CGMP: Questions and Answers, Guidance for Industry.” Center for Drug Evaluation and Research. https://www.fda.gov/media/119267/download (Accessed Aug 6 2020)