PDA Study Explores Role of A.I. in CPV
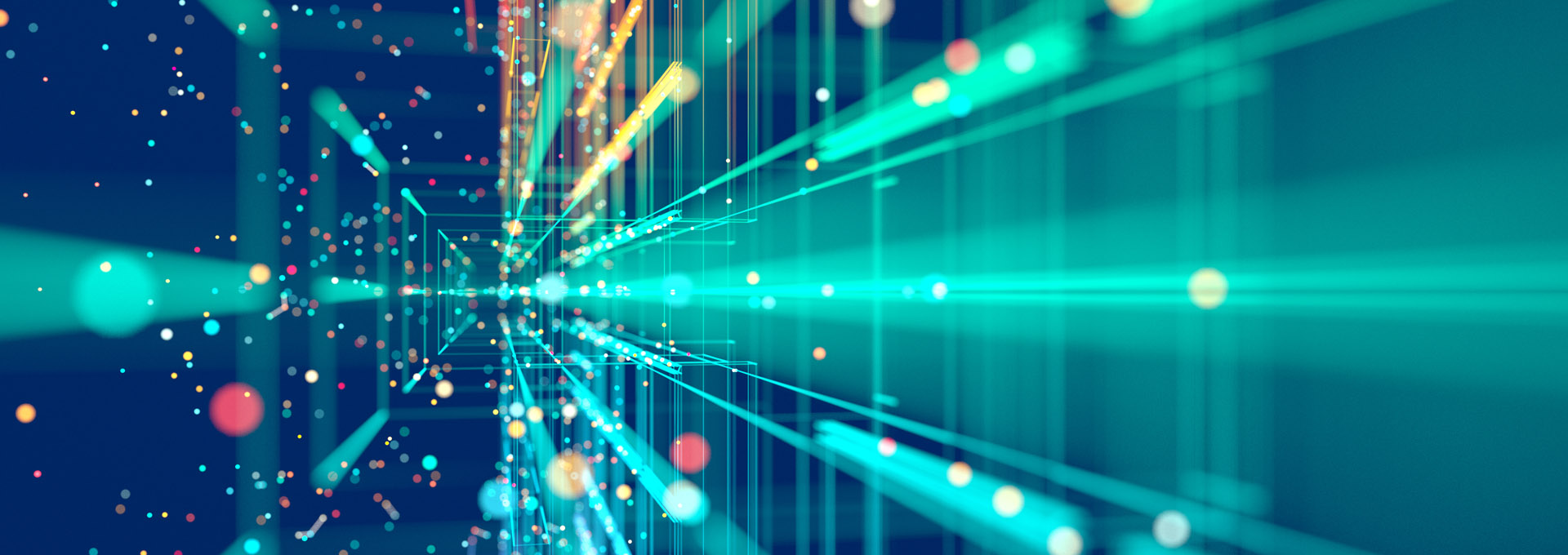
The complexities associated with drug manufacturing is greatly increased with biopharmaceuticals due to the intrinsic variability of the materials involved and the interconnected processes. The control of these multivariate operations must be managed under advanced statistics capable of interpreting complex reactions with multiple factors. The variety of information to be managed and the need to understand the sensitivity of the biosystems suggest that manufacturing in this area could benefit from artificial intelligence (A.I.).
To learn how A.I. could enhance continued process verification (CPV) for biologics, PDA’s Process Validation Interest Group has embarked on an experimental project: CPV of the Future. This project aims to establish a standard procedure for CPV in fermentation operations, applying A.I. as a valid analytical method for process control. This initiative has been designed to explore three distinct phases—Process Design, Continued Process Verification and Process Performance Qualification. The first, Stage 1, focuses on CPV (Figure 1).

In Stage 1, the commercial manufacturing process is defined, based on knowledge gained through development and scale-up. Stage 3 requires continuous assessment, based on Stage 1 principles, in order to ensure the validity of the current factors being considered and process understanding.
This PDA initiative comprises a set of experimental studies that will focus on improving production of the recombined protein generated by the fermentation of the Pichia pastoris microorganism under different conditions of oxygen supply. Specific attention will be paid to hypoxia settings. Using a bioreactor of five-liter volume, fermentations will be performed that produce batches controlled through automatic and manual operations, measuring the following factors:
- Process parameters (pH, temperature, dissolved CO2, dissolved O2, agitator speed, air flow rate, substrate concentration and ethanol concentration)
- KPI – calculated in the laboratory (growth rate, performance indexes, protein concentration, substrate consumption, O2 consumption, CO2 production, respiratory quotient and carbon emission rates)
- Raw materials
- Room and atmospheric conditions
- Manual operations (settings and sampling information)
The first stage of this study will consist of nearly 20 batches with a specific configuration defined by a design of experiments (DoE). This will provide a set of raw data and calculated variables to determine which could be considered critical factors. The analytical strategy is based on a PCA model where the principal components will be used as variables that feed an A.I.-supervised model. Gradient-boosting regressor and random forest regressor are two A.I. algorithms that will be applied in this experiment due to previous success using them in biologics and molecular research (1,2). Both algorithms are machine-learning techniques for regression, which will produce a prediction model in the form of an ensemble of weak prediction models configured as decision trees for this experiment. The known critical quality attribute (CQA) values will determine the acceptance of the A.I. models during the verification phase, while the continuous process data and environmental records will be used to train the models. From the CPV perspective, considering the principal components with an explanatory percentage higher than 80% as predictor factors in the A.I. models is part of the experiment providing innovative vision. The project also includes the principal components as predicted values in real time as well as the rest of the factors considered critical to characterizing the fermentation process. Therefore, the principal components will be calculated in real time and in the same way as the rest of the process parameters identified as relevant for the fermentation.
Based on the guidelines described above, the CPV of the Future project expects to cover three areas of interest:
Biotechnology: Fermentations will produce batches of biomass brought for Pichia pastoris microorganisms. A deeper knowledge of the relevant factors involved in the fermentation of Pichia under hypoxia conditions is expected from this study. Additionally, the inclusion of environment variables and manual operations in the analysis will be considered in order to design subsequent studies for scale-up and technology transfer in larger bioreactors (75 liters to 250 liters).
Technological areas: As an output of this study, a standard procedure will be established that integrates biochemical operations with technologies embraced by Industry 4.0. The Industrial Internet of Things, cloud and serverless infrastructures designed under software-as-a-service architectures, big data aggregation operations and A.I. will drive the electronic information toward so-called “Smart Factory” good practices. A.I. algorithms will be applied using datasets generated by the produced batches in order to obtain A.I. models. The outcome of the proposed A.I. models will focus on various purposes: root cause analysis for batch failures, early detection of wrong batches, critical parameter identification, pattern recognition and critical variables prediction.
Quality control and good practices: Quality control will manage the last tier of the study, based on the continuous process verification concept and the proposal of new standards to apply A.I. as an analytical method in fermentation production. The quality analysis is based on development of a DoE where the critical factors and the A.I. elements are considered in its strategy. Figure 2 illustrates the proposed schema.
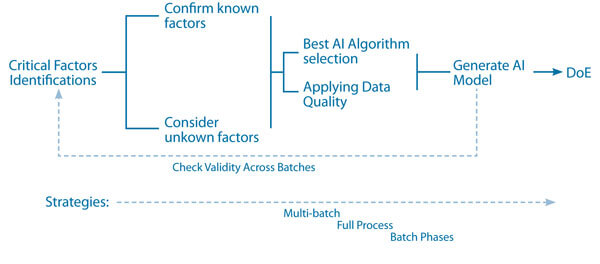
More information on the CPV of the Future project will be presented at the virtual PDA Quality and Regulations Conference, June 9–10.
To learn more and to register, visit the conference website: www.pda.org/EU/QR2020.
Beyond the presupposed benefits presented by the A.I. application in a CPV context, the described requirements to implement the entire framework boast a new way to understand the fermentation process from a multidisciplinary perspective. This project is the beginning of a journey to determine how A.I. techniques will help manufacturers comprehend the complexity inherent to the pharmaceutical processes. PDA will share the results of the study in the future.
References
- Bokulich, N. A., et al. (2018). “q2-sample-classifier: machine-learning tools for microbiome classification and regression.” bioRxiv (Nov. 28, 2018) https://www.biorxiv.org/content/10.1101/306167v2.full
- Wang, R. Significantly improving the prediction of molecular atomization energies by an ensemble of machine learning algorithms and rescanning input space: A stacked generalization approach. J Phys Chem 16 (2018):8868–8873.