FDA/CDER Readying Draft Guidance on AI to Support Regulatory Decision-Making
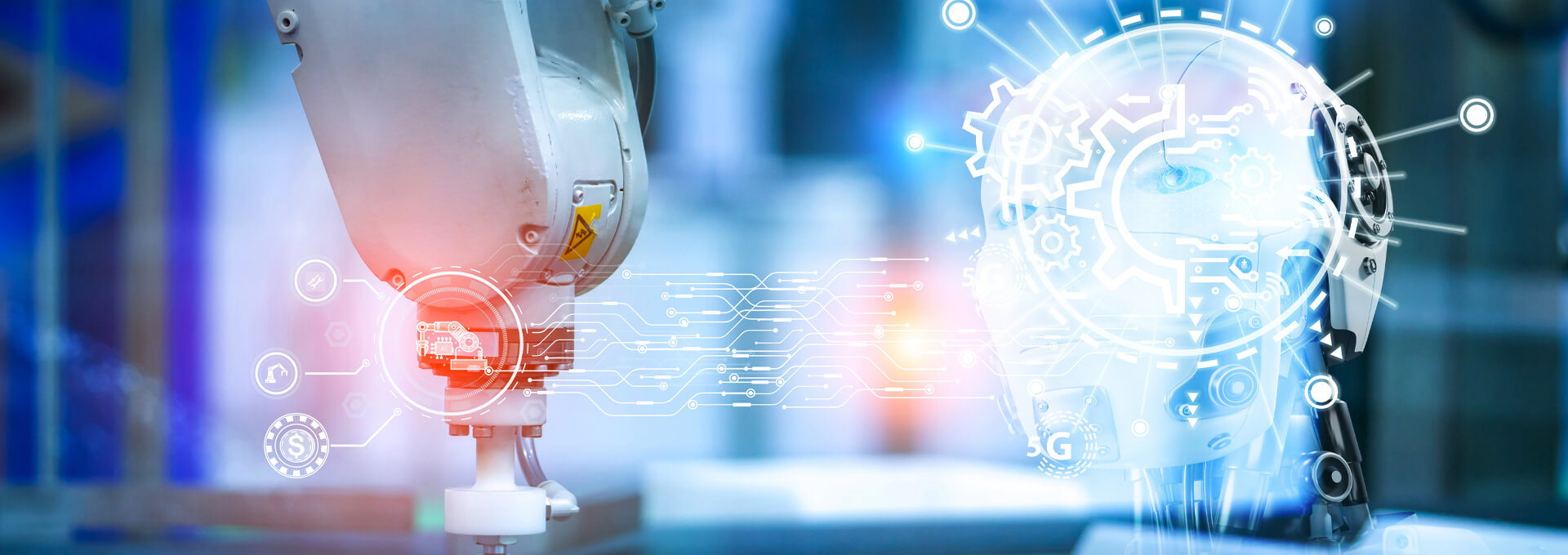
The U.S. FDA Center for Drug Evaluation and Research (CDER) is preparing to release a draft guidance later this year on artificial intelligence (AI), titled Considerations for the Use of Artificial Intelligence to Support Regulatory Decision Making for Drug and Biological Products, according to Tala Fakhouri, Associate Director for policy analysis in CDER’s Office of Medical Policy.
Fakhouri made the announcement during the first day of the Regulatory Education for Industry Annual Conference 2024: CDER (Drugs) Innovation in Medical Product Development (1). Fakhouri mentions that the draft guidance will be based on the FDA’s experience reviewing over 300 submissions regarding AI, over 800 comments received on two 2023 discussion papers, and current regulatory science research.
In 2023, CDER published two discussion papers to elicit industry feedback on AI and machine learning (ML). The first came out in February, entitled Artificial Intelligence in Drug Manufacturing (2). In May, the second discussion paper, Using Artificial Intelligence & Machine Learning in the Development of Drug & Biological Products (3) was released. The initial document discusses the potential applications, benefits and regulatory considerations of using AI and ML technologies in the drug manufacturing process, while also seeking input from stakeholders to assist in understanding the challenges and opportunities in the area. The FDA posed eight specific questions to industry in the document (see sidebar).
[Editor’s Note: Part 1 of this article is Justin Johnson’s examination of the discussion paper on AI/ML in pharmaceutical manufacturing. Part 2 is Walter Morris’s evaluation of selected industry comments on the discussion paper.]
Part 1: A Closer Look into the AI Manufacturing Discussion Paper
Though the discussion paper does not cover certain aspects of AI, like the challenges that could result from the possible obscurity on how to apply existing current good manufacturing practice (CGMP) regulations, it does concentrate on considerations relevant to the manufacturing of drug products intended for market approval through New Drug Applications, Abbreviated New Drug Applications or Biologics License Application pathways.
Based on some of the early feedback that the FDA received from industry members, whether through personal interactions or published content, the document outlines a brief list of examples about how AI might be used in pharmaceutical manufacturing:
- Process design and scale-up: AI models, such as machine learning, generated using process development data could be leveraged to more quickly identify optimal processing parameters or scale-up processes, reducing development time and waste.
- Advanced process control (APC): APC allows dynamic control of the manufacturing process to achieve a desired output. AI methods can also be used to develop process controls that can predict the progression of a process by using AI in combination with real-time sensor data.
- Process monitoring and fault detection: AI methods can be used to monitor equipment and detect changes from normal performance that trigger maintenance activities, reducing process downtime. They can also be used to monitor product quality, including the quality of packaging, for example, vision-based quality control that uses images of packaging, labels or glass vials analyzed by AI-based software to detect deviations from the requirements of a product’s given quality attribute.
- Trend monitoring: AI can be used to examine consumer complaints and deviation reports containing large volumes of text to identify cluster problem areas and prioritize areas for continual improvement. This offers the advantage of identifying trends in manufacturing-related deviations to support a more comprehensive root-cause identification.
Though these are common and practical applications of AI in pharmaceutical manufacturing, the document highlights that considerations are needed when applying such functions of AI in whatever process is being used.
The FDA preposed the following questions in the Artificial Intelligence in Drug Manufacturing discussion paper:
- What types of AI applications do you envision being used in pharmaceutical manufacturing?
- Are there additional aspects of the current regulatory framework (e.g., aspects not listed above) that may affect the implementation of AI in drug manufacturing and should be considered by the FDA?
- Would guidance in AI in drug manufacturing be beneficial? If so, what aspects of AI technology should be considered.
- What are the necessary elements for a manufacturer to implement AI-based models in a CGMP environment?
- What are common practices for validating and maintaining self-learning AI models and what steps need to be considered to establish best practices?
- What are the necessary mechanisms for managing the data used to generate AI models in pharmaceutical manufacturing?
- Are there other aspects of implementing models (including AI-based models) for pharmaceutical manufacturing where further guidance would be helpful?
- Are there aspects of the application of AI in pharmaceutical manufacturing not covered in this document that the FDA should consider?
Areas of Consideration Regarding AI
The discussion paper elaborates five considerations that pertain to certain functions of AI in pharmaceutical manufacturing. These five considerations are centered primarily around the themes of regulatory oversight, and needed industry standards, and clarity when implementing AI.
Regulatory Oversight
According to the document, regulatory oversight appears to be one of the main challenges for the FDA. Cloud applications, for instance, could impact
oversight of pharmaceutical manufacturing data and records. While adopting new cloud- and edge-computing might offer better capabilities for pharmaceutical manufacturing, it also presents challenges in maintaining data integrity, regulatory compliance
and effective risk management, especially regarding third-party services and AI. The discussion paper illustrates this by commenting that “software that controls execution may still be implemented close to the manufacturing equipment to ensure
no impact on performance or security, while other software functions that are not time-critical could occur in the cloud (e.g., model updates, control diagnostics, and process monitoring analytics),” and these tasks are usually done through
third-party data-management systems.
The FDA acknowledges that, though it permits the use of third parties for CGMP functions under proper oversight by the manufacturer, “existing quality agreements between the manufacturer and a third party (e.g., for cloud data management) may have gaps concerning managing the risks of AI in the context of manufacturing monitoring and control.”
In addition, AI systems that continuously learn and adapt to real-time data might also be problematic for regulatory assessment and oversight. The document elaborates on this concept by explaining that, in the current manufacturing paradigm, current models like in-process controls and real-time release-testing undergo development, validation, implementation and updates through the change-control processes of pharmaceutical quality systems. AI models, such as ML-based types, operate differently with continuous-learning capabilities, evolving as new data emerges. Determining when an AI model is established within a process and establishing criteria for notifying regulatory authorities about changes during model maintenance are challenging tasks.
The Impact of the Internet of Things
The increased use of the Internet of Things (IoT) can potentially impact and grow the volume of data generated during the pharmaceutical manufacturing process, including more frequent data recordings and a broader variety of data types. According to the discussion paper, although there are regulations and guidances addressing the amount of data and metadata to be stored for each batch of drug product manufactured, the substantial increase in raw data collected may necessitate balancing data integrity and retention with the logistics of data management. The document suggests there might be a need for clarity concerning regulatory compliance for generated data, for example, “which data needs to be stored and/or reviewed and how the loss of these data would impact future quality decisions such as product recalls.”
Developing and Validating AI Models
Due to the expansion of AI in pharmaceutical manufacturing, there should be industry standards for developing and validating AI models when used for process control and to support release-testing. In this section of the discussion paper, the FDA admits to understanding that AI can be applied in APC to adjust manufacturing processes based on real-time data. Additionally, APC can also support the following:
- Analytical procedures for testing in-process materials or final products
- Real-time release-testing
- Predicting quality attributes of in-process products
Due to the lack of industry standards, however, the FDA believes there could be “challenges in establishing the credibility of a model for a specific use.” Moreover, AI models can also retain knowledge acquired during the development of a specific use-case and then apply it to different, but related use-cases, thereby speeding up model development. Again, the FDA mentions that there needs to be clarity regarding “…how the potential to transfer learning from one AI model to another can be factored into model development and validation.”
Part 2: The Industry Feedback
The discussion paper received 50 comments from the public, 46 of which were made available at the U.S. regulations website (4).
The PDA
PDA commented on the discussion paper on April 28, 2023 (see sidebar for referenced questions) through its standard regulatory commenting process, which involves a drafting task force and approval of the comments by the Science Advisory Board. PDA acknowledged that the discussion paper focused on areas of use for artificial intelligence which are “relevant” and agreed with the development of further regulatory guidance. PDA responded to each of the eight questions posed in the discussion paper.
For question one, PDA identified “quality records,” including deviations, CAPAS, out-of-specification, out-of-trend, and complaints, as the types of AI applications that might be used in pharmaceutical manufacturing. In addition to those, PDA suggested the following systems for specific consideration:
- Manufacturing execution systems
- Packaging operation applications
- Visual inspection
- Digital twins
For question two, on aspects of the current regulatory framework that may affect AI implementation, PDA listed several considerations:
- Robust training
- Clarity on the limits on process control
- Reproducibility of cloud-based AI services
- ALCOA+ principles
- Data extraction from physical records
- Inter-Center coordination within FDA (i.e., CDER and CBER)
- Regulatory harmonization internationally
In question three, the FDA asked about the benefits of guidance on AI for drug manufacturing. PDA recommended the Agency consider other types of documents besides regulatory guidance, such as Q&As, reflection papers and
points-to-consider, “in order to keep pace with rapidly evolving AI.” PDA also stated, “The industry will benefit from a clear definition and example of artificial intelligence. This term is often used broadly to describe digital
technology advancements, many of which are not algorithm or model based.”
As to specific guidance, PDA pointed to a number of aspects of AI and manufacturing:
- Data cleaning and preparation
- Identification of relevant variables, metadata and calculated variables
- Algorithm selection and turning the AI model’s accuracy and performance
- Lifecycle monitoring and continuous supervision of drift within the model
- Traceability and auditability of all steps involved
- Binary classification
- Data management for models using IoT devices
- Change control expectations
Regarding question 8, aspects of AI application not covered in the discussion paper, PDA listed nine:
- Create a communications mechanism between the industry and FDA regarding AI methods in development. Here, PDA recommended conferences, forums, reviews or meetings “where direct access to test and evaluate the models could be provided to expert teams within the Agency.”
- Describe systems and provided guidance around managing AI models in production
- Clarify the difference between “data quality” and “data integrity”
- Specify or identify regulatory pathways for incorporating AI, for example, “Is it required to incorporate AI pathways into Prior Approval Supplements?”
- Clarify any potential advanced capabilities and benefits to organizations adopting AI and consequences, if any, on organizations that do not or cannot adopt AI
- Harmonize with other standards and guidances outside of manufacturing, for example, to include clinical trials and medical devices
- Establish Quality Assurance Agreements between pharma companies and AI service providers
- Clarify FDA’s expectations regarding the United States Pharmacopeia
- Define the risk of “analysis paralysis” due to “‘overthink’ decisions by trending more data than what the simulation or process actually requires.”
PDA’s full comments are available here.
International Consortium for Innovation & Quality in Pharmaceutical Development
The International Consortium for Innovation & Quality in Pharmaceutical Development suggested the following AI applications in pharmaceutical manufacturing among a variety that they listed:
- Automated testing
- Image analysis for online decisions
- AI models through video tagging and classification
- Root-cause analysis
- Batch release
- AI for automated calibration and maintenance models
- AI for predictive stability models
- Projection of design space of new product introduction, “especially on dosage form characteristics from material properties, other inputs and known similar processing operations”
The group saw a number of areas where digital twins could be important, such as manufacturing processes and analytical assays. Another use of digital twin could be for “enabling autonomous platforms (no human intervention), capable of self-optimization, definition of design space and criticality assessment.
The Consortium also highlighted international harmonization as one of many aspects of the current regulatory framework that may affect AI implementation.
As to useful guidance FDA could proffer, the Consortium listed several, including on GMP vs. non-GMP uses of AI, elaboration on “unexplainable ‘black box’ model vs. a potentially acceptable explainable ‘white box’ model,” and “the development, submission, and maintenance of protocols for AI based models [that] would be beneficial and could rely on many elements of ICH Q12.”
Pharmaceutical Research and Manufacturers of America
The Pharmaceutical Research and Manufacturers of America (PhRMA) weighed in with many of its own comments. Before addressing any of FDA’s specific questions, the trade group highlighted several points “to both foster innovation in space and protect public health”. These included:
- Standardized definitions and clarity on regulated aspects of AI
- AI within broader modeling/digital landscape
- International harmonization
- Flexibility in guidance
- Risk-based approach
- Transparency and explainability
In the conclusion, PhRMA said it “strongly supports FDA’s efforts to facilitate innovation in the use of AI in drug manufacturing.”
National Institute for Innovation in Manufacturing Biopharmaceuticals
The 200-member National Institute for Innovation in Manufacturing Biopharmaceuticals (NIIMBL) offered “brief and specific” comments that “do not represent the full scope of comment and concern raised by NIIMBL members but are fully consistent with themes and arcs of member feedback.”
As to what types of AI applications could be used in the pharmaceutical space, NIIMBL stated:
“It is key to distinguish between applications where AI is being used to define the operational space, for example, ‘This is what it looks like when the process runs correctly’ and where AI is being used for control within the operational space, for example, ‘This lot was produced by a process that ran correctly.’ In addition to its role in pharmaceutical development and manufacturing, AI will likely play a role in other parts of the product lifecycle, such as supply and distribution networks to encourage end-to-end quality-by-design approaches.”
Under question three, areas of guidance that would be beneficial, NIIMBL called for guidance on “the mobility of AI technologies between organizations, for example, if Company A acquires or merges with Company B.”
For questions five and six, NIIMBL cautioned: “…we would like to note that common practices for validating and maintaining self-learning AI models and for data management are being developed in a breadth of settings and it is premature to fully identify best practices in biopharmaceutical manufacturing.” The Institute suggested FDA “partner” with the U.S. National Institute of Standards and Technology and provided a link to the NIST AI website.
Amazon Web Services
Amazon Web Services (AWS) issued comments and unsurprisingly focused on cloud services. They agreed with FDA’s assessment of the “critical role of cloud services in drug manufacturing, including as part of hybrid systems where some software executes on hardware close to manufacturing equipment while other software functions and analytics operate remotely.”
As to the role of a cloud service provider, AWS cited “FDA’s allowance for the use of third parties—including cloud service providers—for Current Good Manufacturing Practices,” but requested “additional clarity on the ‘gaps with respect to managing the risks of AI in the context of manufacturing monitoring and control’ called out in the paper.”
AWS continued: “In addition, FDA states in the white paper that these gaps could ‘lead to challenges in ensuring that the third-party creates and updates AI software with appropriate safeguards for data safety and security.’ Additional clarity from FDA on the types of controls and documentation for these controls would help alleviate delays or confusion as part of FDA inspections.”
AWS did not agree with FDA’s statement regarding data traceability and cloud services, stating, “Through the use of [the] cloud, medical product manufacturers may be able to better monitor their manufacturing systems to: identify variance that could affect product quality; secure access to systems and audit changes; and automate processes to result in more consistent, high quality products.”
U.S. Technology Policy Committee
The U.S. Technology Policy Committee (USTPC) also commented on the discussion paper. The Committee advised FDA to “explicitly consider whether AI-based applications should be held to a specialized, more rigorous standard than conventional automated systems.”
With respect to question seven about further guidance that could be helpful, USTPC stated:
“Good governance of AI models requires that they be documented and understood. At minimum, effective governance models would afford regulators access to: information related to use cases, the specific purposes for which a model was developed, model development lifecycle stages, artifacts generated during each step, expert reviews, data lineage, development/deployment platforms, and risk assessments.”
In response to question eight about aspects not covered in the discussion paper, USTPC said: “The FDA, in addition to proposing a risk-based model validation/regulatory framework, should encourage AI developers and pharmaceutical manufacturers to follow principles of Responsible AI when developing and using AI, including those initially developed by USTPC in 2017 as updated in late 2022.”
USTPC urged FDA to consider “a number of its 2019 comments on AI-augmented software.” These novel suggestions are worth reading, among them:
- Removing the human from the “loop,” coupled with bypassing testing, would increase the risk that the AI employed will have unintended effects on data accuracy and device safety.
- There are many possible regulatory responses to these important statistical and physical issues, including:
- Banning… dynamic, significant AI-dictated updates to device behavior because the results cannot be guaranteed accurate and safe
- Requiring a manufacturer to install “governor” software in its device which will reliably assure that the device’s behavior cannot degrade beyond articulable and enforceable limits
- Mandating or encouraging data-sharing among healthcare providers and device manufacturers to maximize the amount, and assure the validity, of large quantities of training data
BioPhorum
The comments from BioPhorum mentioned anticipated reductions in the manufacturing workforce: “Labour: Reduced labour costs with optimized incorporation of Robotics, AGVs, and Cobots.” As to aspects not covered in the discussion paper, BioPhorum mentioned error-free workflow and the laboratory of the future. Regarding the former, the group stated that an error-free workflow consists of monitoring systems that “guide operators, analysts and other GMP critical task without pre-training.” Training in such an environment would occur “through guided workflow and error detection” and would leave “no opportunity for digression or error.”
Biotechnology Innovation Organization
The Biotechnology Innovation Organization (BIO) offered several suggestions to the Agency before delving into the specific questions. Echoing other commenters, the trade group urged the use of International Council for Harmonisation (ICH) guidelines and collaboration across FDA Centers.
Any FDA guidance for AI in manufacturing “should be focused on general principles,” Bio said. They cautioned against “different expectations for different applications of AI-based models in the manufacturing process if they are an equal impact level.”
BIO pointed to Post-Approval Change Management Protocols as the recommended “mechanism to submit, review and maintain models.” The group expressed its belief that “increased frequency in reporting model changes will likely lead to many deviations and ultimately restrict the use of these models.” They also recommended “creation of platform technology master files for third parties to maintain certain proprietary information or processes.
Conclusion
The above comments are just a sampling of those the FDA received in 2023, leading up to the release of the draft guidance expected later this year. Turn back to the PDA Letter for more on this unfolding story as FDA prepares to release the draft guidance.