Deep Learning in the Information Swamp
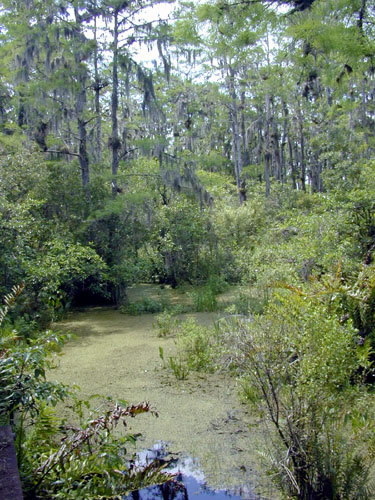
Deep learning. Upon hearing this latest buzzword, you are probably tempted to scream, “No, not another one!” We have already tried artificial intelligence (AI) for quality inspection. Decades ago, we attempted using neural networks, based on rigid decision-making algorithms, to predict or classify certain properties using measurements received from various inspection sensors. Image analysis was also attempted using neural networks. Unfortunately, these networks were built like a black box—signals went in and classifications came out, but nobody really understood how it was accomplished. This made it especially difficult for pharmaceutical quality organizations responsible for validating these systems. No one successfully overcame these difficulties and “black box” neural networks soon disappeared.
Has anything changed since we started using AI? Yes and no. We see an expansion of the Industrial Internet of Things. And we are on the threshold of successful autonomous driving. How can cars discriminate people from lampposts in a fraction of a second, or identify signs and approaching cyclists within the blink of an eye? They do it by using some of the same intelligent algorithms: AI, but advanced AI. Today, innovative machine learning and deep learning tools provide a much stronger basis for more robust recognition rates than in the early years of AI. “Deep learning” is machine learning that enables computers to be trained through complex mathematical structures such as convolutional neural networks (CNN). In simpler terms, a machine’s CNN’s are trained using huge amounts of data that are obtained from images or sensors. Successful training may require 100,000 or more tagged images, comprehensively analyzed by their predefined properties (i.e., labels, tags) and classified accordingly.
The world of automated visual inspection for pharmaceutical products offers many practical benefits. It allows easier discrimination of particles from bubbles, accepts small scratches but rejects severe abrasions and even picks the few oddly shaped forms out of millions of acceptable containers. Deep learning, therefore, is helping machines accurately classify nonconforming units, but with needed flexibility, and at speeds much faster than any human inspector.
For these reasons, deep learning will be a focus of one track at this year’s Visual Inspection Forum, Oct. 23-24 in Berlin. Deep learning may provide the key to capturing the speed and consistency of automated inspection with the intelligence and flexibility of the best human inspectors. Now we just need to address the validation challenges.
Learn more about the 2018 Visual Inspection Forum.