Continued Process Verification: Reacting to Data Signals

With the availability of statistical analysis, modelling tools, and advancements in machine learning and artificial intelligence solutions, the utilization of a growing body of process knowledge gained through the lifecycle stages of process validation is an expectation in the bio/pharmaceutical industry.
The U.S. FDA “Guidance for industry: Process Validation: General Principles and Practices specifies process validation activities in three different stages: Stage 1- Process Design, Stage 2- Process Qualification, Stage 3- Continued Process Verification (CPV) (see Figure 1).
Process validation is no longer a singular finite activity. Stage 3, the Ongoing or Continued Process Verification stage is a formal plan to assure the process remains in its validated state during routine production and the process remains in a state of control. The stage presents various opportunities as well as challenges to the industry. Stage 3 may be best classified into Stage 3a and Stage 3b. The regulatory guidance documents do not delineate Stage 3a and Stage 3b, although the approach is as an industry practice. A robust Stage 3a assessment specifically for understanding and managing process variability provides the basis for a standardized ongoing Stage 3b plan. An effective CPV program would diminish the need for a scheduled/periodic revalidation.

State of Continued Process Verification
With the arrival of Stage 3 (CPV), the expectation is to apply the knowledge gained and to contemporaneously act on the data signals (variability or unexpected patterns in the data) for continuous improvement. This opportunity to analyze the process data more often than in the traditional annual product quality review (APQR) makes the CPV program critical in identifying drifts and proactively eliminating potential process failures.
The early trend detection advantage was one of the reasons for introducing the CPV stage in process validation guidances. The expectation is to apply the CPV insights for new process development and for similar products/processes as well. The CPV stage is expected to generate larger data sets of critical quality attribute (CQA), critical process parameter (CPP), key performance parameter (KPP), and critical material attribute (CMA) since it represents commercial manufacturing phase. Novel technology, data analytics and live data modelling solutions are now available for effective application in CPV programs.
A process validation lifecycle management system with easily accessible Stage 1, 2 and 3 data helps in knowledge management and use of the insights for continuous improvement. Harnessing CPV data is therefore an important business and regulatory need. Emphasis for using a Quality by Design (QbD) approach makes the CPV stage critical for legacy products which were commercialized without QbD (1). Such products may already have a substantial amount of process data for multivariate analysis to establish a design space.
PDA Technical Reports
The industry and regulatory technical guidance continue to evolve for CPV and for the lifecycle approach overall.
PDA technical reports provide sufficient insights on application of the statistical methods and approaches for the process validation lifecycle. For example, PDA Technical Report No. 60: Process Validation: A Lifecycle Approach aligns to the lifecycle process validation model with CPV, as outlined in the 2011 FDA process validation guidance and offers practical examples of process validation lifecycle. PDA Technical Report No. 59: Utilization of Statistical Methods for Production Monitoring present’s relevant and easy-to-use statistical process control (SPC) methods that are applicable to detecting signals in the pharmaceutical/biopharmaceutical industry.
The Gap
Industry organizations like PDA and regulatory agencies have put considerable effort into developing industry friendly and applicable processes for CPV.
Application of standardized sampling plans (e.g., ASTM), SPC signal generation rules, and use of fit for purpose statistical method identified in the technical reports are some examples. They assist in development of the initial CPV program and analyzing the generated process data. However, as the industry matures into continuous improvement decision making, there is a need to apply standardized processes for managing CPV data signals.
To date there are no regulatory guidance on how industry should react to such signals. To fill this gap, the PDA Process Validation Interest Group (PVIG) created the subtopic “Responses to CPV Data Signal”. The teams mandate was to work on rationalizing and standardizing the CPV signal decision making process. The need is imminent since the decisions made based on CPV data signals should not be delayed but also require a high level of statistical and scientific rigor. The decision making cannot be deferred as it impacts the sites operational continuity. The day-to-day CPV decisions once made should stand future regulatory scrutiny.
Another PVIG subtopic team has been exploring the use of CPV data for artificial intelligence applications. The initiative has the support of organizations like the PQRI. The CPV of the future task force is focused on identifying relevant signals which are critical to control ongoing biotech processes.
PVIG: Response to CPV Data Signals
The team, comprised of process validation experts from the biopharmaceutical and pharmaceutical segments, have developed decision-tree models based on brainstorming sessions.
The CPV signals representing CQA/Specification failures are addressed by the quality management system and investigated per standard operating procedures. Out-of-statistical-control (OOSC) and out-of-alert/control are some of limits used for CPV control, which are independent of out-of-specification (OOS) and deviation specification limits.
The scope of project in developing decision-tree model(s) for CPV signal is therefore limited to the data point signals that are out of CPV control limits. The team coined this type of signal as “yellow flags.” Yellow flags represent the process variability and drifts. The FDA process validation guidance is very specific to state that trending should be performed in such a way that it should guard against overreaction to individual events. Thus, automatically categorizing the yellow flags as a quality failure investigation without additional review of the signal is not an ideal approach.
Problems Solved, Opportunity
An upfront approach on how to address the relevant variables and signals is important in avoiding under detection or overreaction. It needs to be noted that the signal can be for a quality attribute, material attribute, process parameter, and/or performance indicator with various degrees of severity.
Examples from biologics and small molecule manufacturing processes were reviewed for applicability. Wherever the term “process” is used in the decision tree, includes analytical methods and any other processes that can be a source of the variability. A CPV program with Stage 3a and Stage 3b delineation would need different approaches since there is not enough data generated in the earlier stages of commercialization (Stage 3a). A decision tree (see Figure 2) was developed for scenarios where there are statistically significant sample sizes.
When limited data is available a deep dive into the available data is warranted to understand what actions can be taken until additional data is generated. A risk-based categorization of signals received (KPP, CPP, CQA etc.) is also required, as all signals are not equally created, leading to requiring different action plans for the yellow flags.
The suggested action (which includes all possible next steps) were determined based on practical relevance and the statistical strength of the signal. For example, it is proposed to take path 1 when the observed drift is a result of an assignable event extrinsic to the process, characterised by an isolated event. This signal may or may not have been identified as a QMS deviation or incident.
Path 2 is suggested when it’s a common cause variation where revision of your control limits may be justified based on current process data. Path 3 is identified for the special cause variations requiring investigation, determination of root cause and continuous improvement remediation and/or a control strategy update. The team’s goal was to develop holistic decision tree examples that can be easily adopted and customized for a product by incorporating product/process specifics.
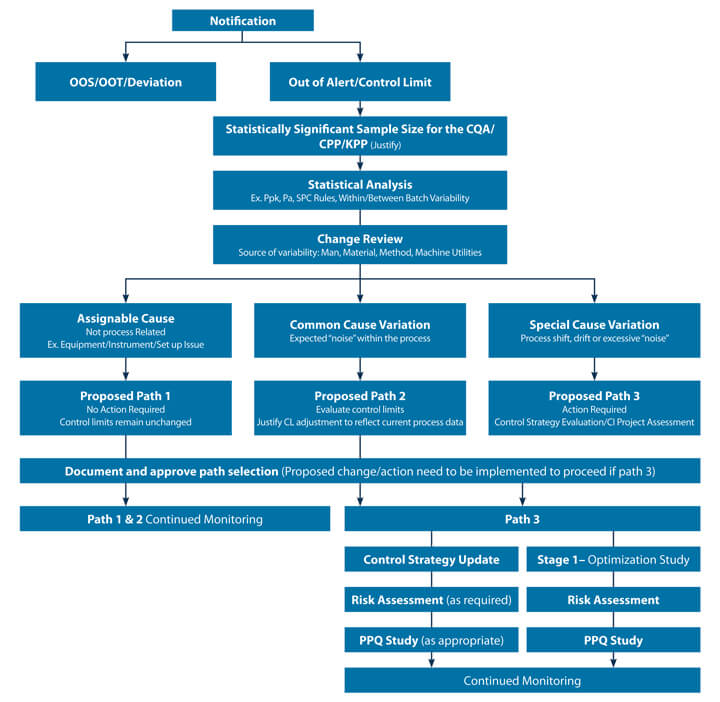
Encouraging Feedback
The efforts of the CPV Subgroup on “Responses to CPV Data Signals” have resulted in developing decision tree flow charts for relevant scenarios. The test cases validated the applicability of the proposed decision trees. A structured decision-making process with grounded statistical rationales minimizes the potential for subjectivity and reduces regulatory compliance risks. The PDA PV subgroup that has developed the process flows invite interested PDA members and industry experts to review and apply the tools on your processes. The feedback and insights garnered will enable the team to further fine tune the tools.
To request more information and for the reaction to signal decision making flowcharts, Contact: [email protected]
References
- U.S. FDA (2009). Q8(R2) Pharmaceutical Development:
https://www.fda.gov/regulatory-information/search-fda-guidance-documents/q8r2-pharmaceutical-development
Responses to CPV Data Signal Subgroup of the PDA Process Validation Interest Group
Ajay Babu Pazhayattil, has been providing strategic direction and leadership is his roles with brand, generic/biosimilar, and CDMO organizations. He is an industrial pharmacist successful in delivering tangible results across segments of biopharmaceutical operations.
Marzena Ingram has more than 19 years of pharmaceutical industry experience leading quality systems and compliance and technical operations teams. Ms. Ingram has worked in drug substance and drug product manufacturing where she developed specialized continued process verification teams and led the implementation of a comprehensive PV life cycle management program. Ms. Ingram serves on the Board of Directors for the Canadian Affiliate of ISPE and has authored multiple pharmaceutical articles.
Aditya Khadilkar is ASQ certified Six Sigma black belt professional having more than 12 years of experience in Pharmaceutical industry. He has worked in the domains such as Manufacturing Science & Technology, Process Engineering Excellence and Validation Statistics. In his current role, he is driving implementation of CPV across the organization, along with digital and analytics tools, to empower data driven decision making.
Bryan K. Schneider is a graduate of the University of Delaware with a Bachelor of Chemical Engineering and MBA. He has held positions in the food, flavors, and pharmaceutical industries working in both R&D and manufacturing. He currently works for Ferring Pharmaceuticals as a Manager in the Process & Operational Excellence group supporting the Parsippany, NJ manufacturing site.
Nienke Vriezen is the Sr. Director of Upstream Development for the Biopharmaceuticals unit at Byondis in Nijmegen. In this capacity she is responsible for the development of cell lines for the production of recombinant proteins, selection and banking of these cell lines, and the subsequent development of the cell culture production process. She also supports technology transfer and process validation across Byondis.
Raphael Bar, formerly at Teva and Pharmos Ltd, is a pharmaceutical consultant for the Pharma industry. He served in the Scientific Advisory Board of PDA.
Mauro Giusti Master degree in Chemistry, Certified QP by MoH, 32 years with Eli Lilly in Pharma Mfg. (roles in Techn Services, Regulatory. Quality, Operations, Project Mngt etc.) Including working for 5 years abroad in USA and UK on assignments. Currently Advisor, Site External Network at Lilly Italia, keeping contacts with Schools, Universities, professional associations, and providing oversight for development of technical capabilities at Lilly Parenteral Products Network level. Part of PDA Italy Chapter for the last 15 years, currently PDA Process Validation Interest Group Co-chair and member of PDA Science Advisory Board.
Toni Manzano is Co-founder and CSO of Aizon. Co-chair of the PDA Biomanufacturing Interest Group, AI Xavier Manufacturing Core Team lead and SME in the Spanish Parliament on big data and artificial intelligence. He teaches Big Data and AI at the UAB University.